Daily Marijuana Use Is Not Associated with Brain Morphometric Measures in Adolescents or Adults
Published in:
Journal of Neuroscience 28 January 2015, 35 (4) 1505-1512; DOI: https://doi.org/10.1523/JNEUROSCI.2946-14.2015
Author Information:
Barbara J. Weiland, Rachel E. Thayer, Brendan E. Depue, Amithrupa Sabbineni, Angela D. Bryan and Kent E. Hutchison
Abstract
Recent research has suggested that marijuana use is associated with volumetric and shape differences in subcortical structures, including the nucleus accumbens and amygdala, in a dose-dependent fashion. Replication of such results in well controlled studies is essential to clarify the effects of marijuana. To that end, this retrospective study examined brain morphology in a sample of adult daily marijuana users (n = 29) versus nonusers (n = 29) and a sample of adolescent daily users (n = 50) versus nonusers (n = 50). Groups were matched on a critical confounding variable, alcohol use, to a far greater degree than in previously published studies. We acquired high-resolution MRI scans, and investigated group differences in gray matter using voxel-based morphometry, surface-based morphometry, and shape analysis in structures suggested to be associated with marijuana use, as follows: the nucleus accumbens, amygdala, hippocampus, and cerebellum. No statistically significant differences were found between daily users and nonusers on volume or shape in the regions of interest. Effect sizes suggest that the failure to find differences was not due to a lack of statistical power, but rather was due to the lack of even a modest effect. In sum, the results indicate that, when carefully controlling for alcohol use, gender, age, and other variables, there is no association between marijuana use and standard volumetric or shape measurements of subcortical structures.
Introduction
The United States has seen changing trends concerning the acceptance of marijuana. As of 2013, 20 states had either decriminalized marijuana or legalized medical use. Colorado, Washington, Oregon, and Alaska have now legalized its recreational use. Concurrently, the popular press has shown significant interest in scientific studies on the effects of marijuana use. Two widely featured studies include one suggesting that regular marijuana use decreases IQ [Meier et al., 2012 (which has been challenged for not accounting for a confounding effect of socioeconomic status); Rogeberg, 2013], and another suggesting that “recreational use” causes brain abnormalities (Gilman et al., 2014).
To be sure, these two studies do not stand alone. Other studies of the relationship between marijuana use and brain morphology have found equivocal results (Lisdahl et al., 2014; Lorenzetti et al., 2014). Marijuana use has been associated with both increased (Cousijn et al., 2012) and decreased (Yücel et al., 2008; Demirakca et al., 2011; Solowij et al., 2011) volumes of subcortical structures, or both (Battistella et al., 2014). Importantly, these studies were not designed to determine causality (i.e., that marijuana use causes morphological changes), which would require a longitudinal design to establish temporal precedence.
Finally, many studies did not adequately exclude the effects of confounding variables. Several reports included marijuana groups that differed from control groups in alcohol use/abuse (Demirakca et al., 2011; Solowij et al., 2011; Schacht et al., 2012; Gilman et al., 2014). Unlike marijuana, alcohol abuse has been unequivocally associated with deleterious effects on brain morphology and cognition in both adults (Sullivan, 2007; Harper, 2009) and adolescents (Nagel et al., 2005; Medina et al., 2008; Squeglia et al., 2012). Statistically controlling for comorbid alcohol abuse, as many studies do, is not an ideal strategy, especially in small groups or under conditions where covariates may interact with the independent variable (Miller and Chapman, 2001). Thus, it is possible that alcohol use, or other factors, may explain some of the contradictory findings to date.
Given the interest in the risks associated with marijuana use among the general public and policy makers, replication of reports that marijuana use is associated with morphological changes in the brain is essential. To that end, we retrospectively examined brain morphology in a sample of adult daily marijuana users (n = 29) versus nonusing control subjects (n = 29), using techniques identical to those used in the study by Gilman et al. (2014). We examined the same variables in adolescent daily users (n = 50) versus nonusers (n = 50). Importantly, there were two differences in our analytic approach. Because the previous study suggested an exposure-dependent effect (Gilman et al., 2014), we compared daily users to nonusers. Evaluating the extremes should provide greater statistical power (McClelland, 1997). Furthermore, groups were matched on the Alcohol Use Disorders Identification Test (AUDIT), whereas groups differed on AUDIT scores in the original article. We evaluated the following structures that were the focus of recent studies of marijuana: the bilateral nucleus accumbens and amygdala (Gilman et al., 2014); hippocampus (Demirakca et al., 2011; Schacht et al., 2012); and cerebellum (Solowij et al., 2011; Cousijn et al., 2012).
Materials and Methods
ADULT PARTICIPANTS AND MEASURES.
Adult participants (N = 503) were recruited from the greater Albuquerque, NM, or Boulder/Denver, CO, metropolitan regions through advertisements for studies on alcohol/substance use. Exclusionary criteria and study details have been specified in previous publications (Filbey et al., 2008; Claus et al., 2011). Written informed consent, approved by the University of New Mexico Human Research Committee, was obtained from all participants.
Participants completed the Time Line Follow Back (TLFB) to assess quantity and frequency of substance use for the past 60 d (Sobell and Sobell, 1992), the AUDIT to assess hazardous drinking/dependence (Saunders et al., 1993), the Impulsive Sensation-Seeking Scale (IMPSS) of the Zuckerman–Kuhlman Personality Questionnaire (Zuckerman et al., 1993), the Beck Depression Inventory (Beck et al., 1961), and the Beck Anxiety Inventory (Beck et al., 1988).
Based on the TLFB data, a subset of subjects was identified as daily marijuana users (n = 29, 16 male and 13 female). From the remaining subjects, age, gender, and AUDIT scores were used to create a matched control group reporting no marijuana use in the past 60 d.
ADOLESCENT PARTICIPANTS AND MEASURES.
Adolescent participants (N = 262) were recruited through juvenile justice services in Albuquerque as part of a larger study on adolescent risk behavior (Magnan et al., 2013). All eligible participants were assented, and parental or legal guardian consent was obtained before participation; the University of New Mexico Human Research Committee approved all study procedures. Exclusionary criteria were the use of psychotropic medications or diagnosis of a psychiatric disorder other than attention deficit hyperactivity disorder.
Adolescents were identified based on the frequency of their marijuana use during the past 3 months (White and Labouvie, 1989) as daily users (n = 50, 41 male and 9 female) or as part of a matched group of nonusers (n = 50, 36 male and 14 female). Additional measures for quantity and frequency of alcohol use and cigarette smoking were obtained from the assessment of the past 3 months (White and Labouvie, 1989). Adolescents also completed the AUDIT and IMPSS as well as the Children's Depression Inventory (Kovacs, 1992).
ANATOMICAL IMAGE ACQUISITION.
Both neuroimaging sites have 3 T Siemens Trio scanners with 12-channel radio frequency coils. High-resolution T1-weighted structural images were acquired using the same 5-echo multi-echo MPRAGE sequence, as follows: TE = 1.64, 3.5, 5.36, 7.22, and 9.08 ms; TR = 2.53 s; TI = 1.2 s; flip angle = 7°; excitations = 1; slice thickness = 1 mm; field of view = 256 mm; resolution = 256 × 256 × 176; voxel size 1 × 1 × 1 mm; pixel bandwidth = 650 Hz.
VOXEL-BASED MORPHOMETRY VOLUMETRIC/DENSITY ANALYSIS.
Voxel-based morphometry (VBM) analyses were performed using the FSLVBM analysis pipeline in FSL (version 5.0.1) (http://fsl.fmrib.ox.ac.uk/fsl/fslwiki/FSLVBM) following standard automated processing (Ashburner and Friston, 2000; Good et al., 2001), as in other publications (Depue et al., 2014; Gilman et al., 2014). Briefly, images were brain extracted and normalized to Montreal Neurological Institute (MNI) standard space. Resulting images were averaged to create a study-specific template, to which native gray matter (GM) images were reregistered and modulated. The modulated segmented images were smoothed with an isotropic Gaussian kernel with a σ of 3, yielding a full-width at half-maximum (FWHM) of 6.9 mm. The resulting subject-specific GM probability maps were input into a general linear model (GLM) to test for group differences between nonusers and daily marijuana users, controlling for intracranial volume (ICV). Two separate GLM analyses were performed to assess the following: (1) whole-brain GM volume/density; and (2) partial volume region of interest (ROI) using the bilateral nucleus accumbens, amygdala, hippocampi, and the cerebellum. Separate masks for each of these seven ROIs were created from the Harvard-Oxford Sub-Cortical Atlas. Multiple-comparison correction used voxelwise thresholding applied using the FSL Randomize permutation-based non-parametric testing with 5000 Monte Carlo simulations. Clusterwise extent correction using the FSL built-in cluster-based thresholding technique was applied with a threshold of t > 2.3.
In addition, we extracted the volume for each of the ROIs; these values were entered into a multivariate GLM (SPSS version 21) to test for group differences, controlling for ICV.
FREESURFER SURFACE-BASED MORPHOMETRY VOLUMETRIC ANALYSIS.
Surface-based morphometry (SBM) analyses used FreeSurfer version 5.1 (https://surfer.nmr.mgh.harvard.edu/) to perform cortical reconstruction and volumetric segmentation were similar to previous work (Gilman et al., 2014; Weiland et al., 2014). Briefly, these methods included motion correction, Talairach transformation, and segmentation and parcellation of cortical and subcortical structures (Dale et al., 1999; Fischl et al., 2004). The resulting subject-specific volume maps were input into GLM analyses to perform whole-brain analyses testing for group differences between nonusers and daily marijuana users, controlling for ICV. To correct for multiple comparisons, p-maps were thresholded to yield an expected false discovery rate of 5% (Genovese et al., 2002). Next, ROI analyses used FreeSurfer output data for bilateral nucleus accumbens, amygdala, hippocampi, and cerebellum. These volumes were entered into a GLM to test for group differences while controlling for ICV.
Finally, FreeSurfer outputs volumetric data for 35 cortical structures per hemisphere, as well as right and left thalamus, pallidum, and the a priori structures tested in the ROI analyses (i.e., nucleus accumbens, amygdala, hippocampus, and cerebellum). Volumes of all 82 structures were entered into a multivariate GLM to test for the group effect on any structure with ICV as a covariate.
FIRST SHAPE ANALYSIS.
Shape analyses were performed using the FSL (version 5.0.1) FIRST toolbox, as in other studies (Depue and Banich, 2012; Depue et al., 2014; Gilman et al., 2014). Briefly, shape models in FIRST are constructed from a library of manually segmented images. FIRST searches for the most probable shape instance given the observed intensities from input images. Segmentation was performed with two-stage transformation to MNI space (Woolrich et al., 2009) with boundary voxels thresholded at 6.9 mm FWHM for bilateral nucleus accumbens, amygdala, and hippocampi (FIRST does not currently provide a shape model for the cerebellum). Permutation testing used FSL Randomize with 5000 Monte Carlo simulations to test for group differences in shape, correcting for multiple vertex comparisons. Clusterwise extent correction was applied, with a threshold of F > 3.0.
EVALUATION OF EFFECT SIZES FROM RECENTLY PUBLISHED PAPERS.
Finally, we sought to compare our study to other recent studies in the literature. We evaluated the articles listed in the recent review by Lorenzetti et al. (2014) and, where volumetric means were available, calculated effect sizes as Cohen's d (Cohen, 1988) for the accumbens, amygdala, hippocampus, and cerebellum.
Results
Participants
Nonusers and daily marijuana users were nearly identical in terms of age and AUDIT scores, with no significant differences on other measures of comorbid alcohol and tobacco use, depression, anxiety, impulsivity, sensation seeking, or education (Table 1).
VBM volumetric/density analysis
The whole-brain and ROI-masked analyses, controlling for ICV, resulted in no clusters meeting significance thresholds between daily marijuana users and nonusers in either the adult or adolescent samples, suggesting that there were no differences between nonusers and daily users in the specific regions of interest or anywhere else in the brain.
The GLMs of extracted ROI volumes found no effect of group on structure volumes; statistics for these GLMs are presented in Tables 2 and 3. To ensure that higher levels of alcohol use were not masking the effects of marijuana use, we repeated the analyses using only subjects with AUDIT scores of <8 (a cutoff considered indicative of problematic use; Saunders et al., 1993) in the adult sample (n = 8 per marijuana group) and the adolescent sample (n = 29 per marijuana group). No effect of group on structure volume was found in either cohort.
FreeSurfer volumetric analysis
The whole-brain analysis, controlling for ICV, resulted in no clusters meeting significance thresholds between daily and nonusers in either the adult or adolescent samples, suggesting that there were no differences between nonusers and daily users in the specific regions of interest or anywhere else in the brain.
The GLMs of extracted ROI volumes found no effect of group on structure volumes; statistics for these GLMs are presented in Table 2. Additional secondary analyses, limited to subjects with AUDIT scores <8 in both the adult and adolescent cohorts, found no effect of marijuana group on structure volumes.
The multivariate GLM evaluating all brain structures found no effect of group in the adults (F(1,53) = 4.783, p = 0.351) or adolescents (F(1,82) = 0.720, p = 0.832) on structure volumes.
FIRST shape analysis
Shape analyses resulted in no clusters meeting the significance threshold in any of the six ROIs evaluated in the adult sample. A significant cluster was found in the adolescent sample in the right hippocampus (x = 63, y = 155, z = 43, k = 1576, t(56) = 6.150, p = 0.040) with a smaller peak scalar value in the daily users [mean (SD): nonusers, −0.0198 (0.2314); daily users, 0.0198 (0.2338)], which would not meet significance with correction for multiple comparisons. Table 3 lists structure statistics for each ROI. Additional secondary analyses, limited to subjects with an AUDIT score <8 in the adult and adolescent samples, found no group effect in shape in either cohort.
Evaluation of effect of marijuana in previous studies
To place our findings in context, we graphed our findings alongside the effect sizes for marijuana users and control groups reported in previous studies. As can be seen in Figure 1, these varied considerably across studies, structures, and hemispheres, with a mean cumulative effect size of d = −0.011, suggesting no effect within the bounds of sampling error.
A, Depiction of subcortical structures evaluated for group differences between daily marijuana users and nonusers. B, Effect size of marijuana use on structure volumes from the current study and recent literature, presented as bilateral structures where available (circle/triangle) or as the entire structure (square). Average values are presented for right and left structures only. NAccFS, Freesurfer; VBM, voxel-based morphometry; L, left; R, right; NAcc, nucleus accumbens; Hpc, hippocampus; Amyg, amygdala.
" data-icon-position="" data-hide-link-title="0" style="-webkit-font-smoothing: antialiased; margin-right: 0px; margin-left: 0px; padding: 8px; border: 0px; outline: 0px; vertical-align: baseline; font-family: inherit; font-size: 0.857rem; font-style: inherit; font-variant-caps: inherit; line-height: inherit; color: rgb(0, 119, 192); display: block; -webkit-box-shadow: rgba(0, 0, 0, 0.14902) 0px 2px 10px 0px; box-shadow: rgba(0, 0, 0, 0.14902) 0px 2px 10px 0px; background-color: rgb(255, 255, 255);">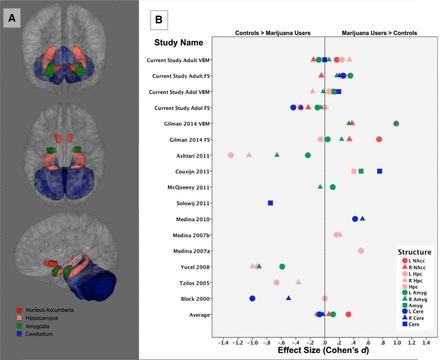
Discussion
Our analyses attempted to replicate previous reports suggesting an exposure-dependent relationship between marijuana use and multimodal measures of brain morphology. The analyses we performed duplicated those previously used (Gilman et al., 2014) with several important differences. Our study included more subjects in adult and adolescent samples, and compared extreme groups of non-marijuana users to daily users. Most importantly, the groups were closely matched on an alcohol problem measure (AUDIT) and were not different on many possible confounding variables (e.g., tobacco use, depression, impulsivity, age, and gender). In other words, the present analyses had greater power to detect group differences, while closely controlling for other effects. We found no evidence of differences in volumes of the accumbens, amygdala, hippocampus, or cerebellum between daily versus nonusers, in adults or adolescents. Moreover, effect size data (Tables 2, 3, Fig. 1) suggest that potential effects are modest and would require very large sample sizes to detect significant differences. The lack of significant differences between marijuana users and control subjects in the present study is consistent with the observation that the mean effect size across previously published studies suggests no clear effect of marijuana on gray matter volumes (Fig. 1). The studies at the top of Figure 1, arguably those with the tightest control over comorbid alcohol use, had the tightest range of effect sizes. Additionally, the top six studies in Figure 1 suggest that choice of analysis software (e.g., FSLVBM or FreeSurfer) impacts effect sizes, highlighting an important consideration when interpreting results in imaging literature and the need to use multiple approaches in data analysis (Gilman et al., 2014).
The present study is one of the only studies to match groups very carefully on a measure of alcohol use severity (i.e., the AUDIT). Unlike the marijuana literature, which has produced somewhat equivocal associations between marijuana use and brain morphology (Lisdahl et al., 2014; Lorenzetti et al., 2014), the literature on the effects of alcohol use on gray matter is unequivocal. Alcohol consumption is associated with volume loss in the brain globally (Harper and Kril, 1985; Jernigan et al., 1991; Pfefferbaum et al., 1992; Hommer et al., 2001; Paul et al., 2008), as well as in specific cortical (Fein et al., 2002; Makris et al., 2008; Durazzo et al., 2011) and subcortical structures, including the caudate nucleus (Jernigan et al., 1991), thalamus (Segobin et al., 2014), amygdala (Fein et al., 2006; Makris et al., 2008), nucleus accumbens (Makris et al., 2008), and cerebellum (Torvik et al., 1986; Sullivan et al., 2000). Studies have also reported that alcohol use is associated with morphological changes in samples of youth drinking below our sample mean (1.3–3.5 drinks/d vs 5.3 drinks/d in this study; Nagel et al., 2005; Medina et al., 2008; Squeglia et al., 2012). Thus, even modest alcohol abuse may be associated with morphological changes and may represent an important confounding variable in studies on the effects of marijuana.
These alcohol findings highlight the need to carefully consider confounding effects of alcohol use. Because alcohol use and marijuana use are often correlated, marijuana groups are also likely to differ on alcohol use. Studies often approach these differences by using alcohol measures as covariates in statistical models. However, this solution may not be adequate, as analysis of covariance when the covariate shares a meaningful relationship to the grouping variable and the dependent measure—undoubtedly the case with alcohol and marijuana use and brain morphology—can lead to inflated effect size estimates and type I error (Miller and Chapman, 2001). Finally, alcohol is not the only potential confounding variable in studies on marijuana. A key difference between marijuana users and control subjects is often the fact that users are willing to engage in a high-risk, illegal behavior while control subjects are not. A major strength of the current adolescent study is that this variable was controlled for, given that nonusers and users were both involved in the justice system (though not incarcerated) and thus had engaged in risky behaviors other than marijuana use. If there is a fundamental biological mechanism underlying “risk,” having similar risky behaviors (e.g., engagement in some form of illegal behavior) in both our adolescent groups should control for any morphological differences related to the willingness to engage in risky behaviors, to allow interpretation of the marijuana effect alone. In support of that logic, these groups did not differ on measures of impulsivity or sensation seeking, placing focus on the difference in marijuana use rather than personality traits.
It is also unclear how variations in the morphology of cortical or subcortical structures would be interpreted. For example, others have interpreted reductions of gray matter volume in the accumbens as evidence of the deleterious effects of alcohol (Makris et al., 2008), yet increases in accumbens volume associated with marijuana use were interpreted as deleterious (Gilman et al., 2014). Future research should link structural differences to behavioral or functional measures to better understand the implications of differences in brain morphology. In addition, the morphological techniques used for analyses show substantial variation in results depending on processing and software, particularly shape analysis (Gao et al., 2014).
Another important issue for future work is the number and variety of chemical components in marijuana. Tetrahydrocannabinol (THC) contributes to the “high” associated with use, but different genetic strains of cannabis may greatly differ on potency of ≥80 additional cannabinoids [e.g., cannabidiol (CBD), cannabinol, cannabigerol, and tetraydrocannabivarin] and terpenoids (e.g., alpha-pinene, myrcene, limonene; Russo, 2007). This is particularly important as CBD has CB1 antagonist properties and may counteract some of the negative effects of THC (Niesink and van Laar, 2013), including hippocampal volume reductions (Demirakca et al., 2011). Ideally, future studies will evaluate and control for cannabinoid content. While that has not been possible to date, it will be possible in states that regulate the sale of marijuana. For example, when an individual purchases marijuana in Colorado, strain and potency information, analyzed by a state-licensed laboratory, is often available, increasing the ability of future research to examine the influence of different cannabinoids and terpenoids.
Multiple other factors will also require attention in future marijuana research, including mode of delivery, which may determine how, and which, cannabis components impact individuals (Abrams et al., 2007); interaction of developmental stage (Lisdahl et al., 2014); and interaction of individual genetics with effects of marijuana on the brain (Caspi et al., 2005; Decoster et al., 2012; Schacht et al., 2012). Longitudinal studies are needed to determine causality rather than associations, to the extent possible with nonexperimental data. Notably, this is a limitation the current study also shares.
Other limitations of our study include group designation based on recent marijuana use rather than detailed history (e.g., age of onset, duration of both marijuana and alcohol use) as well as no inclusion of socioeconomic factors (e.g., maternal drug use, early life stress, nutrition), which may impact brain morphology during development. A number of systematic differences in these factors may have contributed to the group differences found in previous studies (Filbey et al., 2014; Gilman et al., 2014) or masked an effect in this study. In addition, we acknowledge that the adolescents in this study were drawn from a convenience sample of juvenile justice-involved youth who are among the >31 million adolescents under the jurisdiction of the juvenile court system (Office of Juvenile Justice and Delinquency Prevention, 2009). While our results may not generalize to all populations, they would generalize to other youth likely to engage in marijuana use. Finally, it is important to note that the current study does not “prove” that marijuana has no effect on brain morphology. In fact, it is virtually impossible to prove that an effect does not exist. The point of hypothesis testing is not to prove the null hypothesis, but to reject the null hypothesis, which cannot be rejected in this study. Given the well known problems with null hypothesis significance testing writ large (Cohen, 1994; Krueger, 2001), we present effect sizes from the present and previous studies to place our findings in a larger context. We believe this presentation, rather than a singular focus on whether a critical value crosses the significance threshold, is consistent with current recommendations regarding the interpretation of practical and clinical significance of findings across studies (Cumming, 2012).
In conclusion, clear evidence regarding the effects of marijuana on the brain and on health in general are important for informing the public and policy makers about the potential risks and/or benefits of marijuana use. The press may not cite studies that do not find sensational effects, but these studies are still extremely important. While the literature clearly supports a deleterious short-term effect of marijuana on learning and memory (Ranganathan and D'Souza, 2006; Crane et al., 2013), it seems unlikely that marijuana use has the same level of long-term deleterious effects on brain morphology as other drugs like alcohol. It is imperative that rigorous research accurately identifies the harms associated with marijuana use to better inform policy and perception, especially with respect to harm reduction strategies in the face of increasing use.
Footnotes
Published in:
Journal of Neuroscience 28 January 2015, 35 (4) 1505-1512; DOI: https://doi.org/10.1523/JNEUROSCI.2946-14.2015
Author Information:
Barbara J. Weiland, Rachel E. Thayer, Brendan E. Depue, Amithrupa Sabbineni, Angela D. Bryan and Kent E. Hutchison
Abstract
Recent research has suggested that marijuana use is associated with volumetric and shape differences in subcortical structures, including the nucleus accumbens and amygdala, in a dose-dependent fashion. Replication of such results in well controlled studies is essential to clarify the effects of marijuana. To that end, this retrospective study examined brain morphology in a sample of adult daily marijuana users (n = 29) versus nonusers (n = 29) and a sample of adolescent daily users (n = 50) versus nonusers (n = 50). Groups were matched on a critical confounding variable, alcohol use, to a far greater degree than in previously published studies. We acquired high-resolution MRI scans, and investigated group differences in gray matter using voxel-based morphometry, surface-based morphometry, and shape analysis in structures suggested to be associated with marijuana use, as follows: the nucleus accumbens, amygdala, hippocampus, and cerebellum. No statistically significant differences were found between daily users and nonusers on volume or shape in the regions of interest. Effect sizes suggest that the failure to find differences was not due to a lack of statistical power, but rather was due to the lack of even a modest effect. In sum, the results indicate that, when carefully controlling for alcohol use, gender, age, and other variables, there is no association between marijuana use and standard volumetric or shape measurements of subcortical structures.
Introduction
The United States has seen changing trends concerning the acceptance of marijuana. As of 2013, 20 states had either decriminalized marijuana or legalized medical use. Colorado, Washington, Oregon, and Alaska have now legalized its recreational use. Concurrently, the popular press has shown significant interest in scientific studies on the effects of marijuana use. Two widely featured studies include one suggesting that regular marijuana use decreases IQ [Meier et al., 2012 (which has been challenged for not accounting for a confounding effect of socioeconomic status); Rogeberg, 2013], and another suggesting that “recreational use” causes brain abnormalities (Gilman et al., 2014).
To be sure, these two studies do not stand alone. Other studies of the relationship between marijuana use and brain morphology have found equivocal results (Lisdahl et al., 2014; Lorenzetti et al., 2014). Marijuana use has been associated with both increased (Cousijn et al., 2012) and decreased (Yücel et al., 2008; Demirakca et al., 2011; Solowij et al., 2011) volumes of subcortical structures, or both (Battistella et al., 2014). Importantly, these studies were not designed to determine causality (i.e., that marijuana use causes morphological changes), which would require a longitudinal design to establish temporal precedence.
Finally, many studies did not adequately exclude the effects of confounding variables. Several reports included marijuana groups that differed from control groups in alcohol use/abuse (Demirakca et al., 2011; Solowij et al., 2011; Schacht et al., 2012; Gilman et al., 2014). Unlike marijuana, alcohol abuse has been unequivocally associated with deleterious effects on brain morphology and cognition in both adults (Sullivan, 2007; Harper, 2009) and adolescents (Nagel et al., 2005; Medina et al., 2008; Squeglia et al., 2012). Statistically controlling for comorbid alcohol abuse, as many studies do, is not an ideal strategy, especially in small groups or under conditions where covariates may interact with the independent variable (Miller and Chapman, 2001). Thus, it is possible that alcohol use, or other factors, may explain some of the contradictory findings to date.
Given the interest in the risks associated with marijuana use among the general public and policy makers, replication of reports that marijuana use is associated with morphological changes in the brain is essential. To that end, we retrospectively examined brain morphology in a sample of adult daily marijuana users (n = 29) versus nonusing control subjects (n = 29), using techniques identical to those used in the study by Gilman et al. (2014). We examined the same variables in adolescent daily users (n = 50) versus nonusers (n = 50). Importantly, there were two differences in our analytic approach. Because the previous study suggested an exposure-dependent effect (Gilman et al., 2014), we compared daily users to nonusers. Evaluating the extremes should provide greater statistical power (McClelland, 1997). Furthermore, groups were matched on the Alcohol Use Disorders Identification Test (AUDIT), whereas groups differed on AUDIT scores in the original article. We evaluated the following structures that were the focus of recent studies of marijuana: the bilateral nucleus accumbens and amygdala (Gilman et al., 2014); hippocampus (Demirakca et al., 2011; Schacht et al., 2012); and cerebellum (Solowij et al., 2011; Cousijn et al., 2012).
Materials and Methods
ADULT PARTICIPANTS AND MEASURES.
Adult participants (N = 503) were recruited from the greater Albuquerque, NM, or Boulder/Denver, CO, metropolitan regions through advertisements for studies on alcohol/substance use. Exclusionary criteria and study details have been specified in previous publications (Filbey et al., 2008; Claus et al., 2011). Written informed consent, approved by the University of New Mexico Human Research Committee, was obtained from all participants.
Participants completed the Time Line Follow Back (TLFB) to assess quantity and frequency of substance use for the past 60 d (Sobell and Sobell, 1992), the AUDIT to assess hazardous drinking/dependence (Saunders et al., 1993), the Impulsive Sensation-Seeking Scale (IMPSS) of the Zuckerman–Kuhlman Personality Questionnaire (Zuckerman et al., 1993), the Beck Depression Inventory (Beck et al., 1961), and the Beck Anxiety Inventory (Beck et al., 1988).
Based on the TLFB data, a subset of subjects was identified as daily marijuana users (n = 29, 16 male and 13 female). From the remaining subjects, age, gender, and AUDIT scores were used to create a matched control group reporting no marijuana use in the past 60 d.
ADOLESCENT PARTICIPANTS AND MEASURES.
Adolescent participants (N = 262) were recruited through juvenile justice services in Albuquerque as part of a larger study on adolescent risk behavior (Magnan et al., 2013). All eligible participants were assented, and parental or legal guardian consent was obtained before participation; the University of New Mexico Human Research Committee approved all study procedures. Exclusionary criteria were the use of psychotropic medications or diagnosis of a psychiatric disorder other than attention deficit hyperactivity disorder.
Adolescents were identified based on the frequency of their marijuana use during the past 3 months (White and Labouvie, 1989) as daily users (n = 50, 41 male and 9 female) or as part of a matched group of nonusers (n = 50, 36 male and 14 female). Additional measures for quantity and frequency of alcohol use and cigarette smoking were obtained from the assessment of the past 3 months (White and Labouvie, 1989). Adolescents also completed the AUDIT and IMPSS as well as the Children's Depression Inventory (Kovacs, 1992).
ANATOMICAL IMAGE ACQUISITION.
Both neuroimaging sites have 3 T Siemens Trio scanners with 12-channel radio frequency coils. High-resolution T1-weighted structural images were acquired using the same 5-echo multi-echo MPRAGE sequence, as follows: TE = 1.64, 3.5, 5.36, 7.22, and 9.08 ms; TR = 2.53 s; TI = 1.2 s; flip angle = 7°; excitations = 1; slice thickness = 1 mm; field of view = 256 mm; resolution = 256 × 256 × 176; voxel size 1 × 1 × 1 mm; pixel bandwidth = 650 Hz.
VOXEL-BASED MORPHOMETRY VOLUMETRIC/DENSITY ANALYSIS.
Voxel-based morphometry (VBM) analyses were performed using the FSLVBM analysis pipeline in FSL (version 5.0.1) (http://fsl.fmrib.ox.ac.uk/fsl/fslwiki/FSLVBM) following standard automated processing (Ashburner and Friston, 2000; Good et al., 2001), as in other publications (Depue et al., 2014; Gilman et al., 2014). Briefly, images were brain extracted and normalized to Montreal Neurological Institute (MNI) standard space. Resulting images were averaged to create a study-specific template, to which native gray matter (GM) images were reregistered and modulated. The modulated segmented images were smoothed with an isotropic Gaussian kernel with a σ of 3, yielding a full-width at half-maximum (FWHM) of 6.9 mm. The resulting subject-specific GM probability maps were input into a general linear model (GLM) to test for group differences between nonusers and daily marijuana users, controlling for intracranial volume (ICV). Two separate GLM analyses were performed to assess the following: (1) whole-brain GM volume/density; and (2) partial volume region of interest (ROI) using the bilateral nucleus accumbens, amygdala, hippocampi, and the cerebellum. Separate masks for each of these seven ROIs were created from the Harvard-Oxford Sub-Cortical Atlas. Multiple-comparison correction used voxelwise thresholding applied using the FSL Randomize permutation-based non-parametric testing with 5000 Monte Carlo simulations. Clusterwise extent correction using the FSL built-in cluster-based thresholding technique was applied with a threshold of t > 2.3.
In addition, we extracted the volume for each of the ROIs; these values were entered into a multivariate GLM (SPSS version 21) to test for group differences, controlling for ICV.
FREESURFER SURFACE-BASED MORPHOMETRY VOLUMETRIC ANALYSIS.
Surface-based morphometry (SBM) analyses used FreeSurfer version 5.1 (https://surfer.nmr.mgh.harvard.edu/) to perform cortical reconstruction and volumetric segmentation were similar to previous work (Gilman et al., 2014; Weiland et al., 2014). Briefly, these methods included motion correction, Talairach transformation, and segmentation and parcellation of cortical and subcortical structures (Dale et al., 1999; Fischl et al., 2004). The resulting subject-specific volume maps were input into GLM analyses to perform whole-brain analyses testing for group differences between nonusers and daily marijuana users, controlling for ICV. To correct for multiple comparisons, p-maps were thresholded to yield an expected false discovery rate of 5% (Genovese et al., 2002). Next, ROI analyses used FreeSurfer output data for bilateral nucleus accumbens, amygdala, hippocampi, and cerebellum. These volumes were entered into a GLM to test for group differences while controlling for ICV.
Finally, FreeSurfer outputs volumetric data for 35 cortical structures per hemisphere, as well as right and left thalamus, pallidum, and the a priori structures tested in the ROI analyses (i.e., nucleus accumbens, amygdala, hippocampus, and cerebellum). Volumes of all 82 structures were entered into a multivariate GLM to test for the group effect on any structure with ICV as a covariate.
FIRST SHAPE ANALYSIS.
Shape analyses were performed using the FSL (version 5.0.1) FIRST toolbox, as in other studies (Depue and Banich, 2012; Depue et al., 2014; Gilman et al., 2014). Briefly, shape models in FIRST are constructed from a library of manually segmented images. FIRST searches for the most probable shape instance given the observed intensities from input images. Segmentation was performed with two-stage transformation to MNI space (Woolrich et al., 2009) with boundary voxels thresholded at 6.9 mm FWHM for bilateral nucleus accumbens, amygdala, and hippocampi (FIRST does not currently provide a shape model for the cerebellum). Permutation testing used FSL Randomize with 5000 Monte Carlo simulations to test for group differences in shape, correcting for multiple vertex comparisons. Clusterwise extent correction was applied, with a threshold of F > 3.0.
EVALUATION OF EFFECT SIZES FROM RECENTLY PUBLISHED PAPERS.
Finally, we sought to compare our study to other recent studies in the literature. We evaluated the articles listed in the recent review by Lorenzetti et al. (2014) and, where volumetric means were available, calculated effect sizes as Cohen's d (Cohen, 1988) for the accumbens, amygdala, hippocampus, and cerebellum.
Results
Participants
Nonusers and daily marijuana users were nearly identical in terms of age and AUDIT scores, with no significant differences on other measures of comorbid alcohol and tobacco use, depression, anxiety, impulsivity, sensation seeking, or education (Table 1).
VBM volumetric/density analysis
The whole-brain and ROI-masked analyses, controlling for ICV, resulted in no clusters meeting significance thresholds between daily marijuana users and nonusers in either the adult or adolescent samples, suggesting that there were no differences between nonusers and daily users in the specific regions of interest or anywhere else in the brain.
The GLMs of extracted ROI volumes found no effect of group on structure volumes; statistics for these GLMs are presented in Tables 2 and 3. To ensure that higher levels of alcohol use were not masking the effects of marijuana use, we repeated the analyses using only subjects with AUDIT scores of <8 (a cutoff considered indicative of problematic use; Saunders et al., 1993) in the adult sample (n = 8 per marijuana group) and the adolescent sample (n = 29 per marijuana group). No effect of group on structure volume was found in either cohort.
FreeSurfer volumetric analysis
The whole-brain analysis, controlling for ICV, resulted in no clusters meeting significance thresholds between daily and nonusers in either the adult or adolescent samples, suggesting that there were no differences between nonusers and daily users in the specific regions of interest or anywhere else in the brain.
The GLMs of extracted ROI volumes found no effect of group on structure volumes; statistics for these GLMs are presented in Table 2. Additional secondary analyses, limited to subjects with AUDIT scores <8 in both the adult and adolescent cohorts, found no effect of marijuana group on structure volumes.
The multivariate GLM evaluating all brain structures found no effect of group in the adults (F(1,53) = 4.783, p = 0.351) or adolescents (F(1,82) = 0.720, p = 0.832) on structure volumes.
FIRST shape analysis
Shape analyses resulted in no clusters meeting the significance threshold in any of the six ROIs evaluated in the adult sample. A significant cluster was found in the adolescent sample in the right hippocampus (x = 63, y = 155, z = 43, k = 1576, t(56) = 6.150, p = 0.040) with a smaller peak scalar value in the daily users [mean (SD): nonusers, −0.0198 (0.2314); daily users, 0.0198 (0.2338)], which would not meet significance with correction for multiple comparisons. Table 3 lists structure statistics for each ROI. Additional secondary analyses, limited to subjects with an AUDIT score <8 in the adult and adolescent samples, found no group effect in shape in either cohort.
Evaluation of effect of marijuana in previous studies
To place our findings in context, we graphed our findings alongside the effect sizes for marijuana users and control groups reported in previous studies. As can be seen in Figure 1, these varied considerably across studies, structures, and hemispheres, with a mean cumulative effect size of d = −0.011, suggesting no effect within the bounds of sampling error.
A, Depiction of subcortical structures evaluated for group differences between daily marijuana users and nonusers. B, Effect size of marijuana use on structure volumes from the current study and recent literature, presented as bilateral structures where available (circle/triangle) or as the entire structure (square). Average values are presented for right and left structures only. NAccFS, Freesurfer; VBM, voxel-based morphometry; L, left; R, right; NAcc, nucleus accumbens; Hpc, hippocampus; Amyg, amygdala.
" data-icon-position="" data-hide-link-title="0" style="-webkit-font-smoothing: antialiased; margin-right: 0px; margin-left: 0px; padding: 8px; border: 0px; outline: 0px; vertical-align: baseline; font-family: inherit; font-size: 0.857rem; font-style: inherit; font-variant-caps: inherit; line-height: inherit; color: rgb(0, 119, 192); display: block; -webkit-box-shadow: rgba(0, 0, 0, 0.14902) 0px 2px 10px 0px; box-shadow: rgba(0, 0, 0, 0.14902) 0px 2px 10px 0px; background-color: rgb(255, 255, 255);">
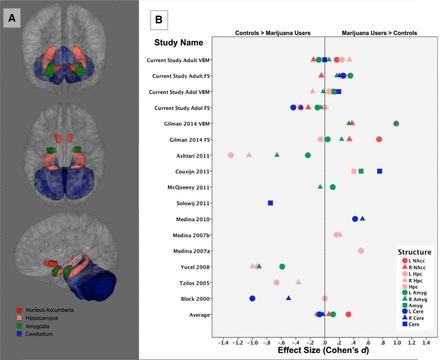
Discussion
Our analyses attempted to replicate previous reports suggesting an exposure-dependent relationship between marijuana use and multimodal measures of brain morphology. The analyses we performed duplicated those previously used (Gilman et al., 2014) with several important differences. Our study included more subjects in adult and adolescent samples, and compared extreme groups of non-marijuana users to daily users. Most importantly, the groups were closely matched on an alcohol problem measure (AUDIT) and were not different on many possible confounding variables (e.g., tobacco use, depression, impulsivity, age, and gender). In other words, the present analyses had greater power to detect group differences, while closely controlling for other effects. We found no evidence of differences in volumes of the accumbens, amygdala, hippocampus, or cerebellum between daily versus nonusers, in adults or adolescents. Moreover, effect size data (Tables 2, 3, Fig. 1) suggest that potential effects are modest and would require very large sample sizes to detect significant differences. The lack of significant differences between marijuana users and control subjects in the present study is consistent with the observation that the mean effect size across previously published studies suggests no clear effect of marijuana on gray matter volumes (Fig. 1). The studies at the top of Figure 1, arguably those with the tightest control over comorbid alcohol use, had the tightest range of effect sizes. Additionally, the top six studies in Figure 1 suggest that choice of analysis software (e.g., FSLVBM or FreeSurfer) impacts effect sizes, highlighting an important consideration when interpreting results in imaging literature and the need to use multiple approaches in data analysis (Gilman et al., 2014).
The present study is one of the only studies to match groups very carefully on a measure of alcohol use severity (i.e., the AUDIT). Unlike the marijuana literature, which has produced somewhat equivocal associations between marijuana use and brain morphology (Lisdahl et al., 2014; Lorenzetti et al., 2014), the literature on the effects of alcohol use on gray matter is unequivocal. Alcohol consumption is associated with volume loss in the brain globally (Harper and Kril, 1985; Jernigan et al., 1991; Pfefferbaum et al., 1992; Hommer et al., 2001; Paul et al., 2008), as well as in specific cortical (Fein et al., 2002; Makris et al., 2008; Durazzo et al., 2011) and subcortical structures, including the caudate nucleus (Jernigan et al., 1991), thalamus (Segobin et al., 2014), amygdala (Fein et al., 2006; Makris et al., 2008), nucleus accumbens (Makris et al., 2008), and cerebellum (Torvik et al., 1986; Sullivan et al., 2000). Studies have also reported that alcohol use is associated with morphological changes in samples of youth drinking below our sample mean (1.3–3.5 drinks/d vs 5.3 drinks/d in this study; Nagel et al., 2005; Medina et al., 2008; Squeglia et al., 2012). Thus, even modest alcohol abuse may be associated with morphological changes and may represent an important confounding variable in studies on the effects of marijuana.
These alcohol findings highlight the need to carefully consider confounding effects of alcohol use. Because alcohol use and marijuana use are often correlated, marijuana groups are also likely to differ on alcohol use. Studies often approach these differences by using alcohol measures as covariates in statistical models. However, this solution may not be adequate, as analysis of covariance when the covariate shares a meaningful relationship to the grouping variable and the dependent measure—undoubtedly the case with alcohol and marijuana use and brain morphology—can lead to inflated effect size estimates and type I error (Miller and Chapman, 2001). Finally, alcohol is not the only potential confounding variable in studies on marijuana. A key difference between marijuana users and control subjects is often the fact that users are willing to engage in a high-risk, illegal behavior while control subjects are not. A major strength of the current adolescent study is that this variable was controlled for, given that nonusers and users were both involved in the justice system (though not incarcerated) and thus had engaged in risky behaviors other than marijuana use. If there is a fundamental biological mechanism underlying “risk,” having similar risky behaviors (e.g., engagement in some form of illegal behavior) in both our adolescent groups should control for any morphological differences related to the willingness to engage in risky behaviors, to allow interpretation of the marijuana effect alone. In support of that logic, these groups did not differ on measures of impulsivity or sensation seeking, placing focus on the difference in marijuana use rather than personality traits.
It is also unclear how variations in the morphology of cortical or subcortical structures would be interpreted. For example, others have interpreted reductions of gray matter volume in the accumbens as evidence of the deleterious effects of alcohol (Makris et al., 2008), yet increases in accumbens volume associated with marijuana use were interpreted as deleterious (Gilman et al., 2014). Future research should link structural differences to behavioral or functional measures to better understand the implications of differences in brain morphology. In addition, the morphological techniques used for analyses show substantial variation in results depending on processing and software, particularly shape analysis (Gao et al., 2014).
Another important issue for future work is the number and variety of chemical components in marijuana. Tetrahydrocannabinol (THC) contributes to the “high” associated with use, but different genetic strains of cannabis may greatly differ on potency of ≥80 additional cannabinoids [e.g., cannabidiol (CBD), cannabinol, cannabigerol, and tetraydrocannabivarin] and terpenoids (e.g., alpha-pinene, myrcene, limonene; Russo, 2007). This is particularly important as CBD has CB1 antagonist properties and may counteract some of the negative effects of THC (Niesink and van Laar, 2013), including hippocampal volume reductions (Demirakca et al., 2011). Ideally, future studies will evaluate and control for cannabinoid content. While that has not been possible to date, it will be possible in states that regulate the sale of marijuana. For example, when an individual purchases marijuana in Colorado, strain and potency information, analyzed by a state-licensed laboratory, is often available, increasing the ability of future research to examine the influence of different cannabinoids and terpenoids.
Multiple other factors will also require attention in future marijuana research, including mode of delivery, which may determine how, and which, cannabis components impact individuals (Abrams et al., 2007); interaction of developmental stage (Lisdahl et al., 2014); and interaction of individual genetics with effects of marijuana on the brain (Caspi et al., 2005; Decoster et al., 2012; Schacht et al., 2012). Longitudinal studies are needed to determine causality rather than associations, to the extent possible with nonexperimental data. Notably, this is a limitation the current study also shares.
Other limitations of our study include group designation based on recent marijuana use rather than detailed history (e.g., age of onset, duration of both marijuana and alcohol use) as well as no inclusion of socioeconomic factors (e.g., maternal drug use, early life stress, nutrition), which may impact brain morphology during development. A number of systematic differences in these factors may have contributed to the group differences found in previous studies (Filbey et al., 2014; Gilman et al., 2014) or masked an effect in this study. In addition, we acknowledge that the adolescents in this study were drawn from a convenience sample of juvenile justice-involved youth who are among the >31 million adolescents under the jurisdiction of the juvenile court system (Office of Juvenile Justice and Delinquency Prevention, 2009). While our results may not generalize to all populations, they would generalize to other youth likely to engage in marijuana use. Finally, it is important to note that the current study does not “prove” that marijuana has no effect on brain morphology. In fact, it is virtually impossible to prove that an effect does not exist. The point of hypothesis testing is not to prove the null hypothesis, but to reject the null hypothesis, which cannot be rejected in this study. Given the well known problems with null hypothesis significance testing writ large (Cohen, 1994; Krueger, 2001), we present effect sizes from the present and previous studies to place our findings in a larger context. We believe this presentation, rather than a singular focus on whether a critical value crosses the significance threshold, is consistent with current recommendations regarding the interpretation of practical and clinical significance of findings across studies (Cumming, 2012).
In conclusion, clear evidence regarding the effects of marijuana on the brain and on health in general are important for informing the public and policy makers about the potential risks and/or benefits of marijuana use. The press may not cite studies that do not find sensational effects, but these studies are still extremely important. While the literature clearly supports a deleterious short-term effect of marijuana on learning and memory (Ranganathan and D'Souza, 2006; Crane et al., 2013), it seems unlikely that marijuana use has the same level of long-term deleterious effects on brain morphology as other drugs like alcohol. It is imperative that rigorous research accurately identifies the harms associated with marijuana use to better inform policy and perception, especially with respect to harm reduction strategies in the face of increasing use.
Footnotes
- This work was supported by the National Institutes of Health through grants from the National Institute on Drug Abuse (K01-DA-031755 to B.J.W., and R01-DA-025074 to K.E.H.) and the National Institute on Alcohol Abuse and Alcoholism (R01-AA-012238 to K.E.H. and R01-AA-017390 to A.D.B.); and by a Brain and Behavior Foundation (NARSAD) Young Investigator Grant (to B.J.W.).
- The authors declare no competing financial interests.
- Correspondence should be addressed to Dr. Barbara J. Weiland, University of Colorado Boulder, MUEN D244, 345 UCB, Boulder, CO 80309-0345. barbara.weiland@colorado.edu
- ↵
- Abrams DI,
- Vizoso HP,
- Shade SB,
- Jay C,
- Kelly ME,
- Benowitz NL
CrossRefPubMedGoogle Scholar - ↵
- Ashburner J,
- Friston KJ
CrossRefPubMedGoogle Scholar - Ashtari M,
- Avants B,
- Cyckowski L,
- Cervellione KL,
- Roofeh D,
- Cook P,
- Gee J,
- Sevy S,
- Kumra S
CrossRefPubMedGoogle Scholar- ↵
- Battistella G,
- Fornari E,
- Annoni JM,
- Chtioui H,
- Dao K,
- Fabritius M,
- Favrat B,
- Mall JF,
- Maeder P,
- Giroud C
CrossRefPubMedGoogle Scholar - ↵
- Beck AT,
- Ward CH,
- Mendelson MM,
- Mock JJ,
- Erbaugh JJ
CrossRefPubMedGoogle Scholar - ↵
- Beck AT,
- Epstein N,
- Brown G,
- Steer RA
CrossRefPubMedGoogle Scholar - Block RI,
- O'Leary DS,
- Ehrhardt JC,
- Augustinack JC,
- Ghoneim MM,
- Arndt S,
- Hall JA
CrossRefPubMedGoogle Scholar- ↵
- Caspi A,
- Moffitt TE,
- Cannon M,
- McClay J,
- Murray R,
- Harrington H,
- Taylor A,
- Arseneault L,
- Williams B,
- Braithwaite A,
- Poulton R,
- Craig IW
CrossRefPubMedGoogle Scholar - ↵
- Claus ED,
- Ewing SW,
- Filbey FM,
- Sabbineni A,
- Hutchison KE
CrossRefPubMedGoogle Scholar - ↵
- Cohen J
- ↵
- Cohen J
CrossRefGoogle Scholar - ↵
- Cousijn J,
- Wiers RW,
- Ridderinkhof KR,
- van den Brink W,
- Veltman DJ,
- Goudriaan AE
CrossRefPubMedGoogle Scholar - ↵
- Crane NA,
- Schuster RM,
- Fusar-Poli P,
- Gonzalez R
CrossRefPubMedGoogle Scholar - ↵
- Cumming G
- ↵
- Dale AM,
- Fischl B,
- Sereno MI
CrossRefPubMedGoogle Scholar - ↵
- Decoster J,
- van Os J,
- Myin-Germeys I,
- De Hert M,
- van Winkel R
CrossRefPubMedGoogle Scholar - ↵
- Demirakca T,
- Sartorius A,
- Ende G,
- Meyer N,
- Welzel H,
- Skopp G,
- Mann K,
- Hermann D
CrossRefPubMedGoogle Scholar - ↵
- Depue BE,
- Banich MT
CrossRefPubMedGoogle Scholar - ↵
- Depue BE,
- Olson-Madden JH,
- Smolker HR,
- Rajamani M,
- Brenner LA,
- Banich MT
CrossRefPubMedGoogle Scholar - ↵
- Durazzo TC,
- Tosun D,
- Buckley S,
- Gazdzinski S,
- Mon A,
- Fryer SL,
- Meyerhoff DJ
CrossRefPubMedGoogle Scholar - ↵
- Fein G,
- Di Sclafani V,
- Cardenas VA,
- Goldmann H,
- Tolou-Shams M,
- Meyerhoff DJ
CrossRefPubMedGoogle Scholar - ↵
- Fein G,
- Landman B,
- Tran H,
- McGillivray S,
- Finn P,
- Barakos J,
- Moon K
CrossRefPubMedGoogle Scholar - ↵
- Filbey FM,
- Aslan S,
- Calhoun VD,
- Spence JS,
- Damaraju E,
- Caprihan A,
- Segall J
Abstract/FREE Full TextGoogle Scholar - ↵
- Fischl B,
- Salat DH,
- van der Kouwe AJW,
- Makris N,
- Ségonne F,
- Quinn BT,
- Dale AM
CrossRefPubMedGoogle Scholar - ↵
- Gao Y,
- Riklin-Raviv T,
- Bouix S
CrossRefPubMedGoogle Scholar - ↵
- Genovese CR,
- Lazar NA,
- Nichols T
CrossRefPubMedGoogle Scholar - ↵
- Gilman JM,
- Kuster JK,
- Lee S,
- Lee MJ,
- Kim BW,
- Makris N,
- van der Kouwe A,
- Blood AJ,
- Breiter HC
Abstract/FREE Full TextGoogle Scholar - ↵
- Good CD,
- Johnsrude IS,
- Ashburner J,
- Henson RN,
- Friston KJ,
- Frackowiak RS
CrossRefPubMedGoogle Scholar - ↵
- Harper C
Abstract/FREE Full TextGoogle Scholar - ↵
- Harper C,
- Kril J
Abstract/FREE Full TextGoogle Scholar - ↵
- Hommer D,
- Momenan R,
- Kaiser E,
- Rawlings R
CrossRefPubMedGoogle Scholar - ↵
- Jernigan TL,
- Butters N,
- DiTraglia G,
- Schafer K,
- Smith T,
- Irwin M,
- Grant I,
- Schuckit M,
- Cermak LS
CrossRefPubMedGoogle Scholar - ↵
- Kovacs M
- ↵
- Krueger J
CrossRefPubMedGoogle Scholar - ↵
- Lisdahl KM,
- Wright NE,
- Kirchner-Medina C,
- Maple KE,
- Shollenbarger S
CrossRefPubMedGoogle Scholar - ↵
- Lorenzetti V,
- Solowij N,
- Fornito A,
- Lubman DI,
- Yucel M
CrossRefPubMedGoogle Scholar - ↵
- Magnan RE,
- Callahan TJ,
- Ladd BO,
- Claus ED,
- Hutchison KE,
- Bryan AD
PubMedGoogle Scholar - ↵
- Makris N,
- Oscar-Berman M,
- Jaffin SK,
- Hodge SM,
- Kennedy DN,
- Caviness VS,
- Marinkovic K,
- Breiter HC,
- GasicGP,
- Harris GJ
CrossRefPubMedGoogle Scholar - ↵
- McClelland GH
CrossRefGoogle Scholar - McQueeny T,
- Padula CB,
- Price J,
- Medina KL,
- Logan P,
- Tapert SF
CrossRefPubMedGoogle Scholar- Medina KL,
- Schweinsburg AD,
- Cohen-Zion M,
- Nagel BJ,
- Tapert SF
CrossRefPubMedGoogle Scholar- Medina KL,
- Nagel BJ,
- Park A,
- McQueeny T,
- Tapert SF
CrossRefPubMedGoogle Scholar- ↵
- Medina KL,
- McQueeny T,
- Nagel BJ,
- Hanson KL,
- Schweinsburg AD,
- Tapert SF
CrossRefPubMedGoogle Scholar - Medina KL,
- Nagel BJ,
- Tapert SF
CrossRefPubMedGoogle Scholar- ↵
- Meier MH,
- Caspi A,
- Ambler A,
- Harrington H,
- Houts R,
- Keefe RS,
- McDonald K,
- Ward A,
- Poulton R,
- Moffitt TE
Abstract/FREE Full TextGoogle Scholar - ↵
- Miller GA,
- Chapman JP
CrossRefPubMedGoogle Scholar - ↵
- Nagel BJ,
- Schweinsburg AD,
- Phan V,
- Tapert SF
CrossRefPubMedGoogle Scholar - ↵
- Niesink RJ,
- van Laar MW
CrossRefPubMedGoogle Scholar - ↵
- Office of Juvenile Justice and Delinquency Prevention
- ↵
- Paul CA,
- Au R,
- Fredman L,
- Massaro JM,
- Seshadri S,
- Decarli C,
- Wolf PA
CrossRefPubMedGoogle Scholar - ↵
- Pfefferbaum A,
- Lim KO,
- Zipursky RB,
- Mathalon DH,
- Rosenbloom MJ,
- Lane B,
- Ha CN,
- Sullivan EV
CrossRefPubMedGoogle Scholar - ↵
- Ranganathan M,
- D'Souza DC
CrossRefPubMedGoogle Scholar - ↵
- Rogeberg O
Abstract/FREE Full TextGoogle Scholar - ↵
- Russo EB
CrossRefPubMedGoogle Scholar - ↵
- Saunders JB,
- Aasland OG,
- Babor TF,
- de la Fuente JR,
- Grant M
CrossRefPubMedGoogle Scholar - ↵
- Schacht JP,
- Hutchison KE,
- Filbey FM
CrossRefPubMedGoogle Scholar - ↵
- Segobin SH,
- Chételat G,
- Le Berre AP,
- Lannuzel C,
- Boudehent C,
- Vabret F,
- Eustache F,
- Beaunieux H,
- Pitel AL
CrossRefPubMedGoogle Scholar - ↵
- Sobell LC,
- Sobell MB
Google Scholar - ↵
- Solowij N,
- Yücel M,
- Respondek C,
- Whittle S,
- Lindsay E,
- Pantelis C,
- Lubman DI
CrossRefPubMedGoogle Scholar - ↵
- Squeglia LM,
- Sorg SF,
- Schweinsburg AD,
- Wetherill RR,
- Pulido C,
- Tapert SF
CrossRefPubMedGoogle Scholar - ↵
- Sullivan EV
CrossRefPubMedGoogle Scholar - ↵
- Sullivan EV,
- Deshmukh A,
- Desmond JE,
- Mathalon DH,
- Rosenbloom MJ,
- Lim KO,
- Pfefferbaum A
CrossRefPubMedGoogle Scholar - ↵
- Torvik A,
- Torp S,
- Lindboe CF
CrossRefPubMedGoogle Scholar - Tzilos GK,
- Cintron CB,
- Wood JB,
- Simpson NS,
- Young AD,
- Pope HG Jr.,
- Yurgelun-Todd DA
CrossRefPubMedGoogle Scholar- ↵
- Weiland BJ,
- Korycinski ST,
- Soules M,
- Zubieta JK,
- Zucker RA,
- Heitzeg MM
CrossRefPubMedGoogle Scholar - ↵
- White HR,
- Labouvie EW
Google Scholar - ↵
- Woolrich MW,
- Jbabdi S,
- Patenaude B,
- Chappell M,
- Makni S,
- Behrens T,
- Beckmann C,
- Jenkinson M,
- Smith SM
CrossRefPubMedGoogle Scholar - ↵
- Yücel M,
- Solowij N,
- Respondek C,
- Whittle S,
- Fornito A,
- Pantelis C,
- Lubman DI
CrossRefPubMedGoogle Scholar - ↵
- Zuckerman M,
- Kuhlman DM,
- Joireman J,
- Teta P,
- Kraft M
CrossRefGoogle Scholar